The Researchers' Zone:
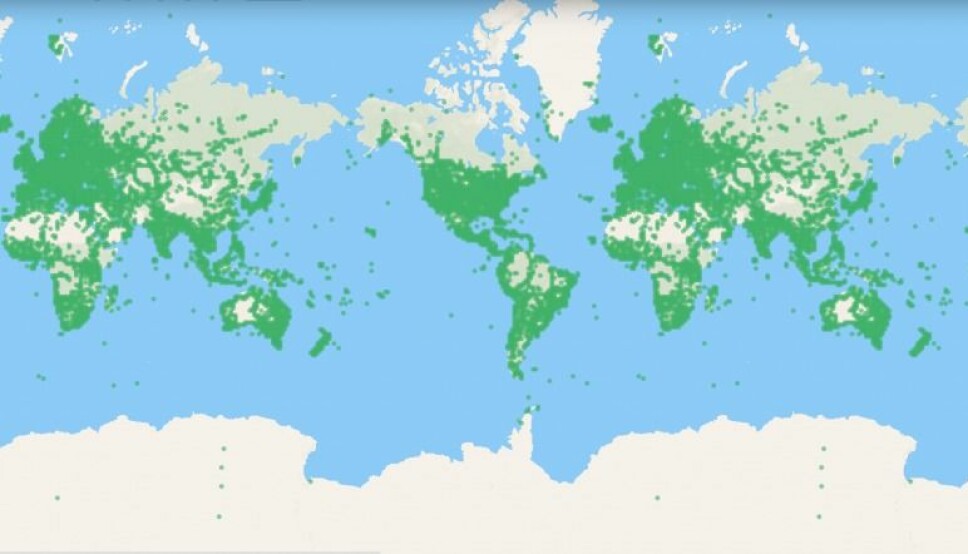
The future map of the world is all about power and money – and it risks making the world more unequal
COMMENT: Future maps of roads and cities will be made by an artificial intelligence that may very well have only seen a small part of the world. This can result in a geo-cultural bias that can make the world more unequal.
We all know the feeling well. It is easier to orientate yourself in a new city in your own country than in a new Asian or African city.
Instinctively, you know that once you have found the square, and when the buildings become old, you are at the centre.
The same is true when we utilise artificial intelligence. Artificial intelligence also recognises places that follow the same architectural and cultural patterns that it has seen before.
This is because we (and artificial intelligence) have learnt to identify the objects on the street plan that we need to pay special attention to and can use as markers to recognise a place.
For example, an advertisement on a neon sign may be a very useful landmark in Copenhagen, but it would be less useful for a specific place in Tokyo.
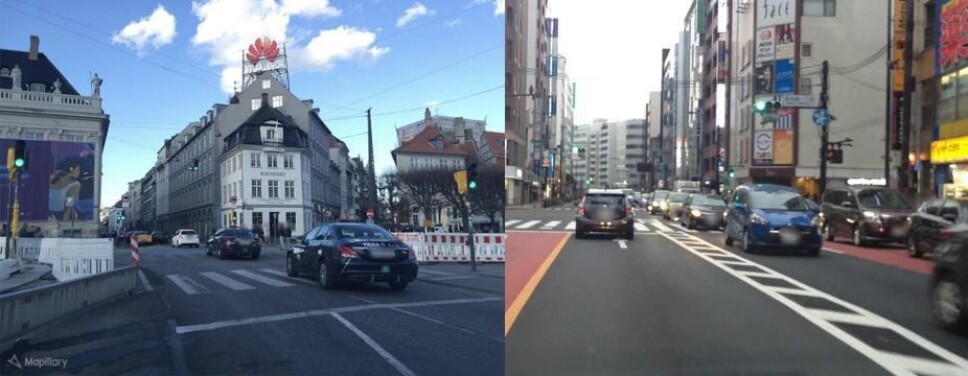
In our research at the Technical University of Denmark (DTU), we have discovered that the artificial intelligence used to recognise places utilises the same behaviour.
This can have undesirable consequences, such as creating greater inequality and discrimination in the maps of the future.
We have taken on these consequences by assembling the world's largest public data set for place recognition with images of the world's cities. We will return to this later.
Maps of the future are 3-dimensional
Since the release of GPS for civilian purposes and the proliferation of map services like Google Maps, we have witnessed a revolution in the use and precision of maps.
Modern maps are dynamic and can update us on rush hour traffic, store opening hours and road closures.
But there is still a lot of research into improving maps. Existing maps do not contain enough information for near-future applications such as self-driving cars and augmented reality (AR - a technology that combines physical data with virtual data; for example, with graphics and sound).
Self-driving cars and AR need far more informative maps than those available now. Self-driving cars will, for example, rely on maps with information on the location of traffic signs, pedestrian crossings, vegetation such as shrubs and trees, road quality and road width.
The maps of the future will be 3-dimensional rather than 2-dimensional and function as a digital copy of reality.
Artificial intelligence making progress
Maps with such a high level of detail require automisation. Fortunately, artificial intelligence has, over the past decade, made great progress in many of the disciplines that are important for making the maps of the future.
For example, artificial intelligence is now able to recognise traffic signs, traffic lights, vegetation and buildings from images.
It can distinguish between roads, road markings, pedestrian crossings, pavements and curbs. And it can make precise 3D reconstructions of the street plan.
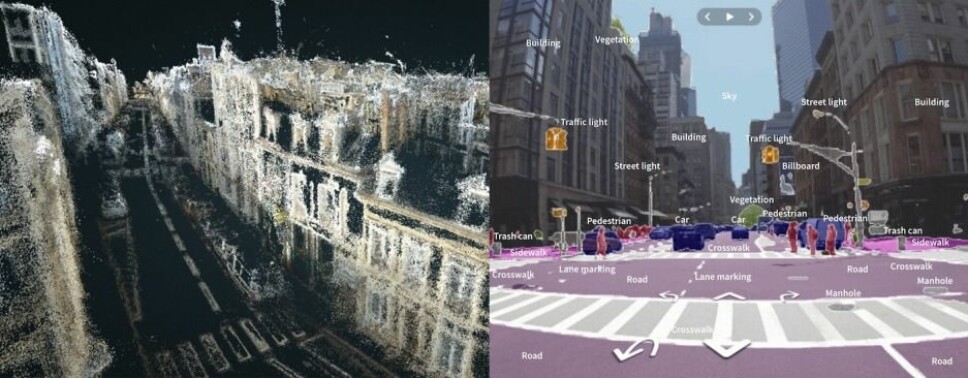
Geographical and cultural bias in maps
In our research at DTU Compute, we are working on an artificial intelligence that can identify the position of a self-driving car based solely on images of the car's surroundings.
We can do this by looking for descriptive features in the street scene that are informative for a specific place but are also visible in many places.
For example, the Round Tower is a very descriptive landmark of a place in Copenhagen – but everywhere else in Denmark or abroad it will not be helpful to look for the Round Tower.
In Copenhagen, descriptive features could be balconies, columns or cobblestones. but an artificial intelligence specialised in looking for balconies, columns or cobblestones will have difficulty orienting itself in Bangalore, India, as there are other visual characteristics here, such as palm trees or billboards, that are descriptive and often appear in the cityscape.
Because we have less data from African and Asian cities, our artificial intelligence has not seen enough examples to learn which visual characteristics are both descriptive and often seen in these areas.
This introduces a bias (skewing): in less represented places, like African and Asian cities, fewer useful pointers will be remembered.
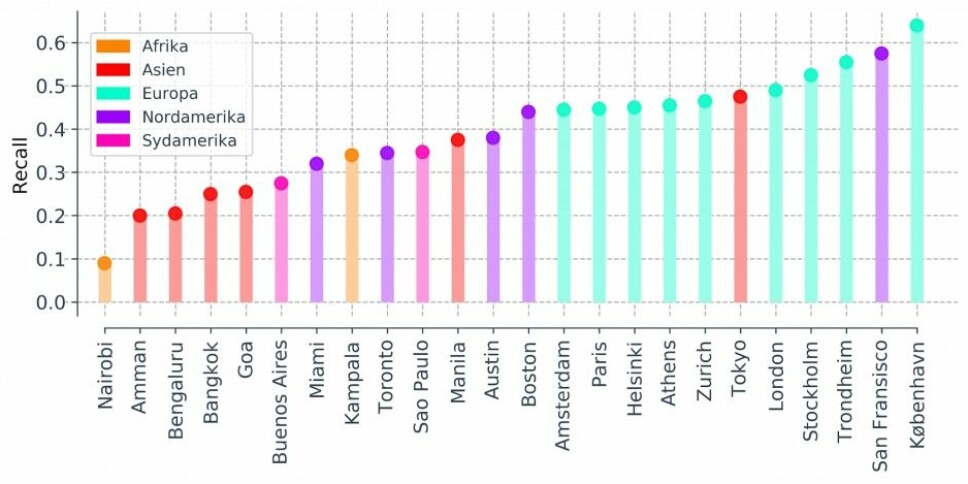
Future maps may be flawed in developing countries
We have seen in our experiments that geo-cultural bias results in artificial intelligence performing much better in cities built on a Western model.
When artificial intelligence is less accurate in other cities, it is not only because we have less information about these places but also because the artificial intelligence is trained to recognise visual characteristics that are descriptive of places in American and European cities.
This bias may result in the advanced digital maps of the future being flawed in African and Asian regions.
This could mean, for example, that self-driving cars and advanced drones will not be able to be used in these areas, resulting in increased global technological and economic disparities.
Car sharing in developing countries requires future maps to be without bias
The most likely scenario is that self-driving cars will become as widespread as shared cars.
In many developing countries, the population density is low. Many have long distances to school or work, and few can afford to buy their own car.
Here, share-friendly, self-driving cars can replace the need for ownership. This will lower the cost of procurement, maintenance and fuel, which in turn will allow more people to have access to transport.
Thus, self-driving cars provide a real opportunity for developing countries to skip the stage of development where they own private means of transport and go directly to a share-friendly society instead.
But to achieve this, geo-cultural bias in future 'intelligent' maps need to be reduced.
Artificial intelligence is trained in Western data
Artificial intelligence is trained in massive amounts of data from Western cities but little or no data from the rest of the world.
By collecting more data from African and Asian cities, these places will also be represented in our datasets, thereby reducing the models’ bias.
Today, companies like Google and Uber are leading the way in the maps of the future through extensive private research into self-driving cars.
However, as there is the greatest demand for these cars in the West, more data is clearly collected in Western, industrialised countries than in developing countries.
This creates an inequality and can lead to technical failures if self-driving cars, which are only trained to recognise distinctive features from European and American cities, are used in African and Asian cities where useful markers look different.
Good maps provide power and strategic infrastructure
Maps have been associated with power throughout the ages. In the Renaissance, land and sea maps were often state secrets as they gave the bearer economic, military and diplomatic advantages.
In modern times, the NAVSTAR GPS system (today just GPS) was originally developed for military purposes. The U.S. government first made GPS available for civilian purposes globally in 2000, 22 years after the GPS system's launch.
In the near future, self-driving transport will become widely used. But all these self-driving means of transport will depend on precise, 3-dimensional copies of reality.
This means that the owners of the data and of the intelligent maps can control all self-driving transport. This would be like the privatisation of the use of the road network.
The best solution to prevent a few companies becoming the only ones that possess these maps would be to democratise access and contribution to them.
Collaborative map services provide an opportunity for this.
Shared maps where everyone can contribute
Companies like Mapillary and OpenStreetMap offer such a collaborative software service, where non-specialists can help develop maps.
For example, anyone can take and upload photos to Mapillary's collaborative platform. Here, your images, along with images from thousands of others, become part of a detailed visualisation that will eventually cover the whole world.
This gives anyone with a camera the ability to contribute to a common map.
Both Mapillary and OpenStreetMap have been very successful in mapping the world, and, at the time of writing, Mapillary has over five million users and over one billion images on their platform.
As a result, large parts of the world are represented in their data, providing more equal opportunities for everyone to map.
The dream of democratic maps made us collect 1.6 million images
We believe it is important that researchers use data from such collaborative platforms in their research as this provides an opportunity to assess the behaviour of artificial intelligence across borders and geo-cultural biases.
This is precisely what we have done on a large scale, and we are the first to do so.
In collaboration with Mapillary, we have created the largest publicly available dataset for location recognition to date, with over 1.6 million images from 30 cities spread across 6 continents (see Figure 2).
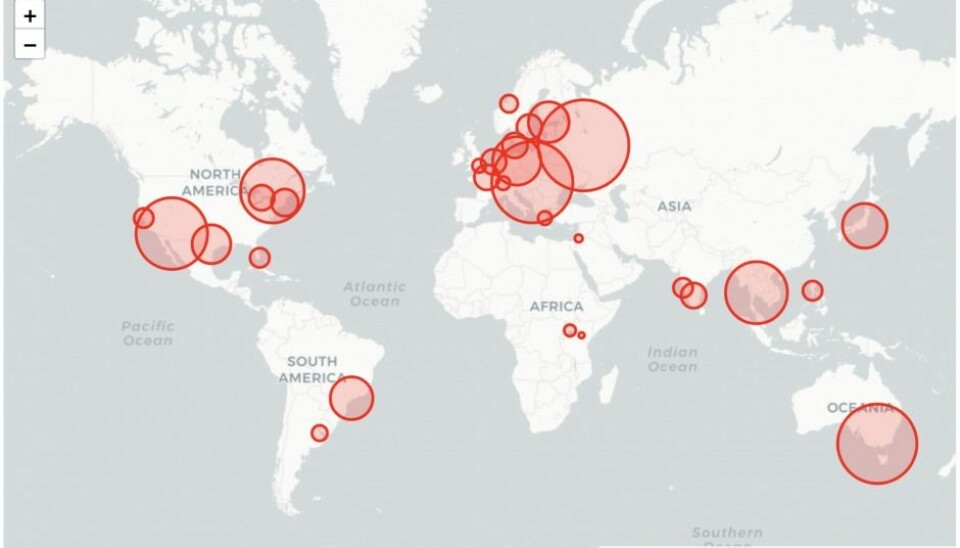
We have compiled a dataset with enormous diversity that represents geo-cultural features across cities like Copenhagen, Kampala, Bengaluru and Tokyo, and we have shown that the dataset can be used to evaluate geographic bias and reduce it.
These results are to be presented at the 'Computer Vision and Pattern Recognition' conference in July 2020, and the corresponding article can be found online here.
Our hope is that our work can help democratise intelligent maps – so that all citizens of the world can benefit from them.
The research on which this article is based has been carried out in part with the help of funds from the Villum Foundation and the European Research Council (ERC).
Translated by Stuart Pethick, e-sp.dk translation services. Read the Danish version at Videnskab.dk's Forskerzonen.
References
Frederik Warburg’s profile (DTU)
———