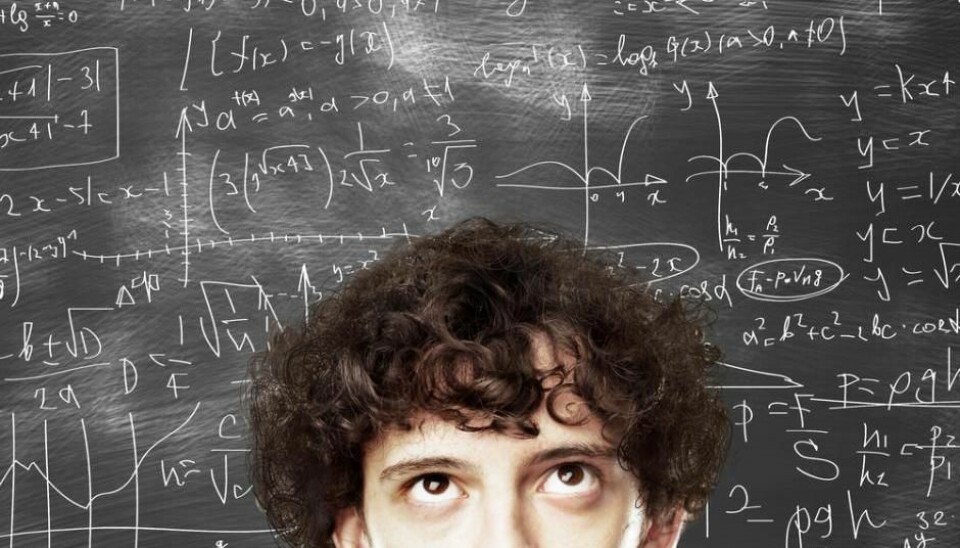
P-values--abused but not abandoned
This statistical tool plays a central role in analysing data, but it is under attack.
One afternoon in the south of England circa 1919, the biologist Ronald Fisher poured a cup of tea and handed it to his colleague Miss Muriel Bristol. She declined, saying that she preferred the taste of tea, when the milk had been poured before the tea, and not the other way around.
She insisted that she could taste the difference prompting a third person, William Roach, to suggest a blind tasting. Afterwards, Roach enthusiastically declared that Muriel could, in fact, tell the difference.
He may not have been entirely objective (he later married Muriel), and the details surrounding Muriel’s achievement are, unfortunately, undocumented. But the episode with the tea had a long-lasting impact on science.
It prompted Ronald Fisher, a gifted mathematician, to develop the basic principles for statistical analyses--principles that are still used widely by scientists today.
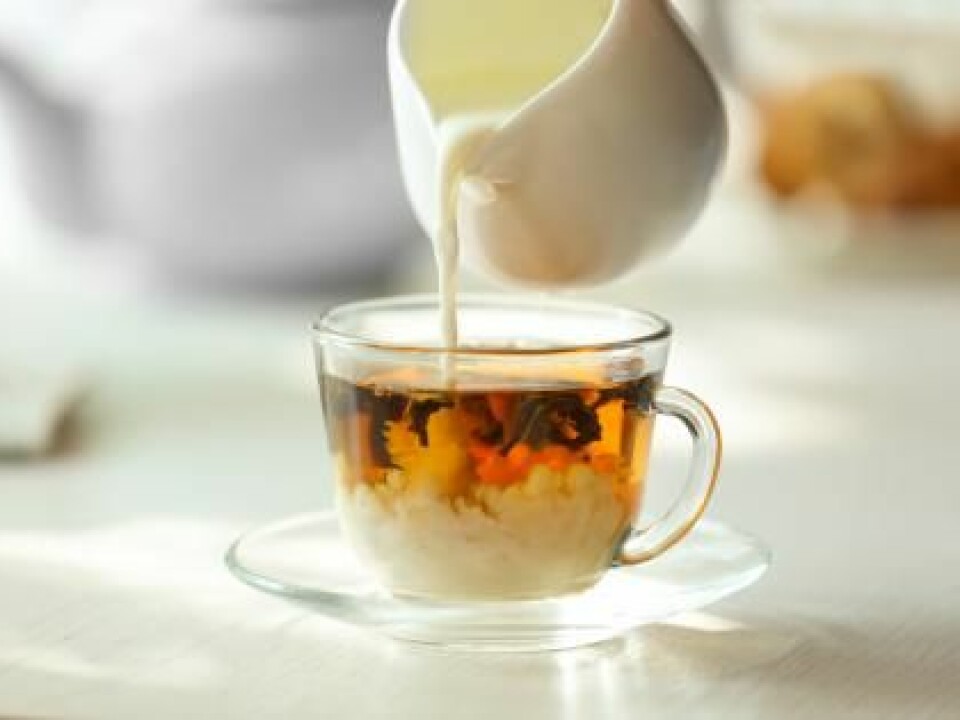
Read More: Statistics might save us from resistant bacteria
Science based on tea
The tea experiment was the starting point of a famous examination in Fisher’s book, ‘The Design of Experiments’.
He pictured a scenario where there are eight cups. In four of them, the milk was poured first, in the last four the tea was poured first. The eight cups are presented to Muriel in random order. Now the question is: if she correctly points out the four cups, in which the milk has been poured first, can we then conclude that she is actually able to identify the order in which the drinks were mixed?
Fisher reasoned that if Muriel could not taste the difference, but merely guessed (the null hypothesis) then he could calculate the probability that she would correctly identify the four cups in the experiment by chance.
The result is 1/70 or about 1.5 per cent. Since this probability is small, we can reject the null hypothesis that Muriel was simply guessing, and recognise Muriel’s impressive tea tasting skills.
The 1.5 per cent is called the p-value or probability value. Simply put, the p-value generally reflects the probability of achieving the observed test result if the null hypothesis is true.
Read More: New maths to predict dangerous hospital epidemics
An integrated part of quantitative research
The p-value is interesting because its calculation is probably the most used, most misunderstood, and most criticised procedure in modern experimental research, and has big financial, societal, and human consequences.
Here is a more scientific sounding scenario:
A research team measures the ability of a new drug to lower blood pressure in a group of people with hypertension. At the same time, they measure the effect of a known drug in a control group. If the new drug works better than the old drug in the experiment, can we then be certain that this applies to the patient population in general?
The null hypothesis is conservative and predicts that the two drugs work equally well. Due to random variation, you could still observe differences between the drugs. But if the probability for this--the p-value--is low, then we can reject the null hypothesis and conclude that the new drug works best (i.e. we disregard the possibility that the old drug is best).
But a high p-value would suggest that the observed difference could easily have occurred by chance and that the two drugs are in fact equally good. In that situation, you would accept the null hypothesis that the effect is the same for both drugs.
But how small should the p-value be before we reject the null hypothesis?
Of course, there is not a fixed boundary. Fisher drew the line at 1/20 (five per cent). This convention is still widely used, for example in medical science. The result of an experiment is considered ‘significant’ when the p-value is less than five per cent, and the entire procedure of calculating the p-value and checking that it crosses this boundary is called a ‘significance test’.
Read More: No math gene: learning mathematics takes practice
Classic misinterpretation of the p-value
The p-value is commonly misinterpreted. Fisher’s hypothetical-deductive approach is straightforward, but there are countless examples where even the researchers themselves get it wrong.
If you find a stronger effect of the new drug with a p-value of four per cent, many people might subtract 4 from 100 and conclude, “The experiment showed a whopping 96 per cent probability that the new drug works best.”
But the probability does not directly relate to the assumption that the new drug is best. It concerns the observation (the difference in effect during the test) under the assumption that the effect of the two drugs is actually the same.
One statistician compared it with confusing the two questions: “Is the Pope a Catholic?” and “Is a Catholic the Pope?” The answer to the first question is “yes,”, whereas the answer to the second question is “unlikely.”
The correct conclusion is: “If the new and old drug worked equally well, the probability of randomly observing the effect recorded during the experiment (or an even bigger difference) would be four per cent.”
Read More: Economists: Too little transparency in research
The p-value must also be analysed
A common point of criticism is that scientists are overly reliant on the p-value compared to the many other quantitative measures that are available to summarize experimental results.
The size of the observed effect is especially important. Does the new drug work only slightly better or much better? Even though a very low p-value strongly implies that the new drug is better, the difference in effect can easily be too small to have any practical importance .
Besides, a p-value less than five per cent is not a complete guarantee of a difference in effect. As long as the p-value is not extremely low, the result could still have occurred randomly even though there is no real difference (the p-value is the size of that risk by definition).
You can, on the other hand, risk reporting a p-value higher than five per cent, thereby maintaining the null hypothesis that both drugs work the same--even though there actually is a difference. This could occur if the experiment is not sensitive enough because there are too few test subjects--in technical terms, the experiment lacks power.
Overlooking a more efficient new drug is of course unfortunate, but it is also unfortunate to falsely conclude that the new drug does not have worse side effects than the old, based on an insignificant p-value.
The official authorities that approve the introduction of new drugs are expected to be aware of these errors. But it is important to note that the risk of making them is an inevitable problem, which follows from the core principles of significance tests.
Read More: Basic research crisis? Many results cannot be replicated
P-value hacking
Many scientists think that the conventional significance level of five per cent has gained an unreasonable level of importance. Numerous researchers have first committed to using the five per cent criterion, only to calculate a higher p-value later--for example eight per cent.
This can lead to despair as it forces them in principle to maintain the null hypothesis. In the example above, the conclusion would be that the new drug is no better than the old one. This can be a great disappointment when much time and money has been invested in the hope of a difference. Often, a 'non-significant' p-value (above 5 per cent) is considered an uninteresting outcome that is unlikely to be published.
In this situation, some scientists argue that eight per cent is not so far from five per cent, and use phrases such as “nearly significant” or “in reality significant.” A humorous list of more than 500 examples of similarly creative expressions have been compiled by Matthew Hankins from University of Southampton.
Another common reaction is to recalculate the p-value using different statistical models, hoping to get below the five per cent--a dubious practice known as p-hacking. Others resort to filtering their data to achieve the desired level of significance.
These issues have implications for another well-known problem in the world of science: the lack of publication of negative results, even though they can be very informative. We will delve into this further in part two.
---------------
Read this article in Danish on Forskerzonen, part of Videnskab.dk
Translated by: Caroline Burchardt