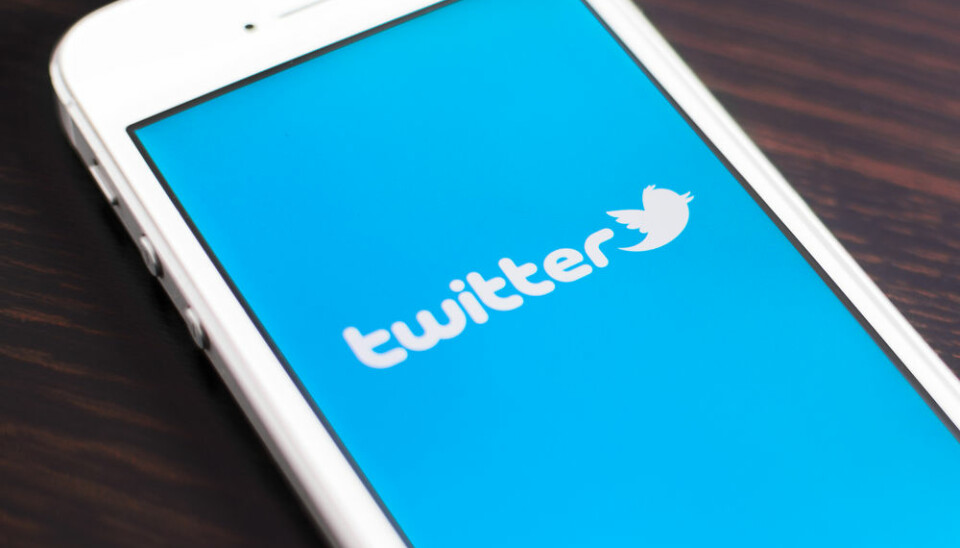
Your tweets reveal future iPhone sales
Researchers set new standards for how precisely the sale of a product can be predicted based on what people write in the social media.
"My iPhone 6 will be sent to my house in less than a week", "I'm really considering whether I should keep my iPhone 5S or whether to have an iPhone 6 & my stepfather asks me every single day" and "So, yet another iPhone charger has disappeared."
These are three random tweets about Apple's iPhone sent during the few minutes before the first sentences of this article were written.
At first sight, they look like simple personal tweets about everyday life, but on the basis of this kind of short message, researchers at Copenhagen Business School (CBS) are now able to predict with considerable precision just how many iPhones will be sold in the near future.
"This information is very important for companies if it can help them predict sales," says Ravi Vatrapu, professor of human computer interaction and head of the Computational Social Science Laboratory at CBS.
Vatrapu studies how to work with Big Data, the enormous dataset being made available to an increasing extent to authorities and businesses.
In this connection he met two students, Niels Buus Lassen and René Madsen, both of whom already had 10 years of experience working with data.
The three researchers have just published a method which can convert a stream of tweets into a crystal ball which can compete with the kind they have in large investment banks. Their results was recently presented at the EDOC 2014 conference in Germany.
500 million tweets about iPhones
The team's reason for choosing the iPhone as opposed to another product is the fact that the Apple product has been one of the most talked about in the world for years. Since 2007, 500 million tweets have contained the word iPhone and a good many are added to that number every minute.
Of course, the world is a complex place, and just because somebody has written about iPhones on their Twitter profile or Facebook it does not necessarily mean they’re going to buy one. So why the correlation between tweets and iPhone purchases?
"We say that tweeting about an iPhone shows that a user is aware of its existence. We don't know whether the user is an existing customer, a future customer or simply a robot sending automatic messages about iPhones, but that’s not important to us," says Vatrapu. “What’s important is that the user is aware of the product and that this awareness will always be at one of four stages.”
The researchers base their method on a sales model known as AIDA (Awareness, Interest, Desire and Action) which claims everyone goes through four phases before purchasing a product.
-
The first phase is that you become aware of the product. This may be an iPhone you have seen on the train, read about in the newspaper -- or seen in a tweet.
-
Then follows phases 2 and 3, in which you first become interested in the product and then start to prefer it in relation to the competition, e.g. by reading about it or asking friends for advice on social media.
-
In phase 4 you finally take action: by making a purchase -- or deciding to keep your old phone.
20 days from tweet to sale
The researchers add up the number of tweets without considering whether the writer is in phase 1, in which he or she is aware of the product (which is difficult to do if you are not) or whether they are further into the process -- because all phases leads to making a purchase or finally deciding not to buy: whether you write about having just discovered the existence of the iPhone and are therefore in phase 1, or you write that you have just dropped your iPhone into the lavatory and intend to buy a new one, putting you in phase 4.
While the latter example is likely to result in to a purchase within a few days, the Twitter user who has just discovered the existence of the iPhone is likely to take a few days getting through the phases before making a purchase.
Vatrapu says it’s possible to precisely predict purchases in a three-month period on the basis of tweets made up to 60 days before that three-month period, although the most precise correlation arises with tweets 20 days before.
"The social consequences of tweets about sales in the real world spread over 20 days. This is not theoretical, it’s empirical – we’ve discovered that the most unambiguous correlation between tweets and sales arises after 20 days. Both before and after this, the correlation is weaker," says Vatrapu.
By looking at tweets 20 days before the beginning of a three month period, the researchers can predict sales within an error margin of five per cent.
"That's about as precise as you can get,” says Vatrapu.
The researchers recently predicted that Apple would sell 36.2 million phones in the three-month period before the iPhone 6 came on the market -- which is more or less the same as the industry's own prediction.
“The great thing about this kind of social science is that we are either right or mistaken, and we can see how mistaken we’ve been when Apple publishes its sales figures," he says.
He emphasises that the model tells them not about the individual Twitter user or iPhone buyer, but that the correlation is between the overall volume of tweets and overall sales.
Setting new standards
This study of data from the social media is by no means the only one of its kind. Earlier studies were able to predict earnings from major Hollywood moving pictures extremely precisely from tweets about the films during the week prior to the first showing and during the first two weeks in the cinemas. However, according to Vatrapu, the trio from CBS have done more to fine tune the model than anyone before them.
"We’ve built on the existing models and improve them by taking into account things such as what time of the year the messages are sent. This is something I believe could improve all prediction models," he says
One reason the researchers correct the figures to take into account the time of year during which the Twitter messages were written is that sales in Western countries increase around Christmas time -- whereas they fall during the period before October. The same period Apple usually launches its new iPhones.
However, while buyers wait for the new iPhone to arrive, talk of it in the social media may well increase -- a factor the model is built to take into account. The model also examines how positive and negative messages are.
"But there’s potential for making significant improvements,” says Vatrapu. “Right now there is an empirical correlation between tweets and sales, which we can explain theoretically using the AIDA model, but now we’ve started looking into how we can improve the model.”
To do this the researchers will examine whether it is possible to analyse which of the four phases of the AIDA model a tweet belongs to -- and discard all the tweets that belong to phase 4 and say that the user has just bought a phone and which thus belongs to sales in the past rather than the future.
Difficult to use on Minor products
Associate professor Sune Lehmann of the Department of Mathematics and Computer science at Denmark's Technical University (DTU) finds the CBS researchers' result interesting. He studies how we make use of the social networks such as Twitter.
"What they've done looks very reasonable to me," says Lehmann but points out that the Twitter research focuses mainly on major products such as the iPhone.
"Their research isn't entirely different from what has been done before -- they're still based on Huberman," says Lehmann with reference to an article published in 2002, in which Bernardo A. Huberman of Hewlett-Packard Laboratories predicted film incomes on the basis of social media data. The CBS researchers also cite Huberman in their new study.
"Huberman's method has proven to be problematic -- for example when applied to less popular phenomena,” says Lehmann. “His methods work for the hit movies he discusses in the article, but things get more difficult when it comes to Indie films.”
“The question is whether the method can be generalised in something that would be of interest to Danish companies," he says.
Translated by: Hugh Matthews
Scientific links
- 'Predicting the future with social media'. Applied Energy, 2013. DOI: 10.1016/j.apenergy.2013.03.027