Researchers' Zone:
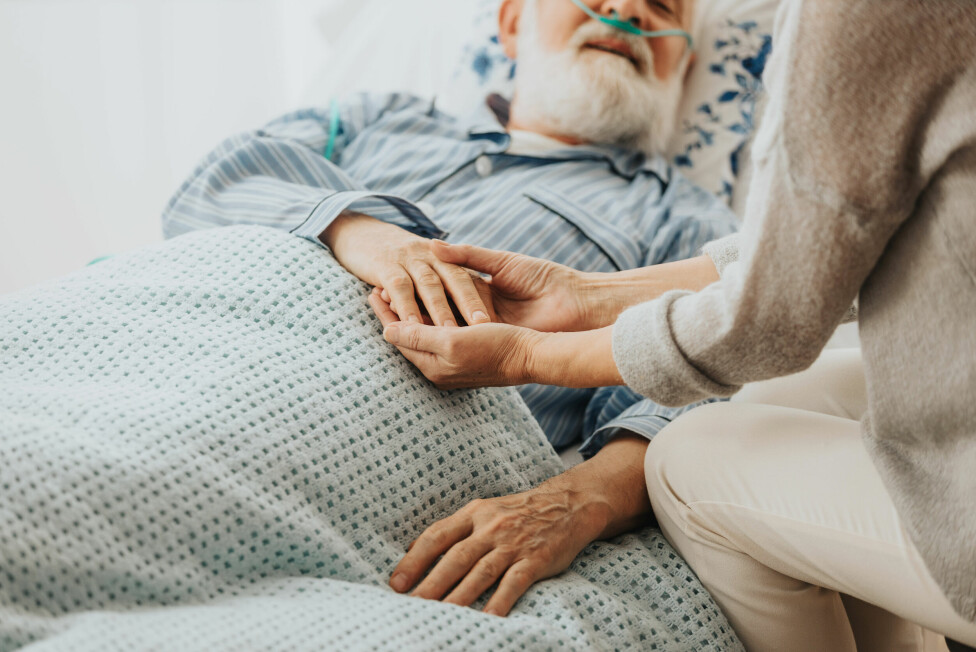
Can AI help limit harm to patients with terminal cancer?
Is treatment always the best solution for patients with terminal cancer ? Researchers exploring if AI can help doctors make a better decision.
The treatment of cancer patients has made tremendous progress in the last decades.
Some patients, even with metastatic disease, can be cured, or can be living for decades with a cancer under control.
This progress has been made possible by the optimization of existing techniques but also by the development of innovative approaches such as immunotherapy and targeted treatments.
Nevertheless, for most of the patients, their cancer will either not be sensitive to these innovative treatments or become resistant after some time.
Traditional anti-cancer treatments (chemotherapy) become then the last resort, but they come with frequent and potentially severe side effects.
This leaves a big responsibility on the doctors to determine that a treatment will not do more harm than good.
Our goal is to investigate how artificial intelligence (AI) can help doctors make these difficult decisions more objectively.
Side effects degrade life quality
Anti-cancer treatments, especially traditional ones, come with frequent side effects such as fatigue, nausea, or diarrhoea and can lead in some occasions to life-threatening conditions such as infection.
These side effects degrade the quality of life of the patients during treatment with the hope to improve their health in the longer term by killing the cancer cells.
But if the patient is too weak and dies during the treatment or shortly after, giving these drugs would not have helped the patient but on the contrary further degraded their quality of life in their last moments.
Will the patient always benefit from treatment?
In a previous study, we have shown that for up to 20 percent of treatments with traditional drugs such as gemcitabine for pancreas cancer, the patient dies less than a month after the end of the treatment.
The challenge is that it is very difficult to predict well in advance when a patient will die, and doctors tend to be too optimistic concerning the survival of their patients.
With experience, doctors will acquire a better sense of this, but it can be difficult to communicate to other doctors, patients, and relatives.
AI can predict the risk of death
In the meantime, Artificial Intelligence (AI) has made major progress in the last years, as illustrated by chatGPT and DALL-E 2, making it always more efficient at identifying complex patterns to predict events.
AI seems therefore well suited to help doctors decide whether chemotherapy or palliative care is the best solution by making more precise predictions of each patient’s death risk.
In a recently published study, we have compared different AI-approaches to predict the risk of death within 30 days for lung cancer patients.
We have shown promising results with the possibility to reduce the number of drug administrations in the last month for these patients by up to 40 percent.
2000 cases trained the AI models
To reach these results, we have collected a large quantity of data on more than 2000 lung cancer patients that died between 2009 and 2019 in the North Denmark Region.
The data included previous diseases, notably chronic ones, blood tests, pathological results, and previous treatment information.
These data were used to train AI models to make predictions of 30-day mortality as accurate as possible at any time point during the patient treatment.
We have tested different AI models to identify the best performing one and evaluated the potential benefit of using such a tool in a clinical context.
Explaining the predictions, a challenge
A major aspect in the development of such solution is the ability to explain the prediction to doctors.
Indeed, AI models can often be black boxes for which it is challenging to understand predictions.
In some cases, it can even lead to inadequate decision of stopping treatment that could be detrimental to the patient survival.
In our study, we included a method to make the AI model more explainable by assessing the importance of each variable in a prediction.
This gives a way for doctors to evaluate the validity of the prediction, and potentially learn from it.
Next step: Will it work in practice?
The next step is to implement a functional prototype at the hospital to conduct a research study.
Our goal is to ensure the AI-tool works as intended and, while having no negative impact on the survival of patients, can limit the usage of these unnecessary drugs near of the end of life of cancer patients.
Ideally, this should lead to an earlier referral to palliative care.
If this study can confirm the performance of the AI-tool, notably that it does not lead to stopping treatment too early, we will work on implementing a proper AI-tool to be used by doctors in their daily work.
It will be initially put into service in Region North Denmark, but we hope to expand its use in the future to other Danish and European regions.
Our hope is that it will allow to limit the harm to cancer patients in their last moments, for example to be able say goodbye to their family in better conditions.
This article was originally published in Danish on our sister site, Forskerzonen.
References
- Charles Vesteghem's profile (AAU)
- Rasmus Froberg Brøndum's profile (AAU)
- 'Thirty-Day Mortality Following Systemic Anticancer Therapy: Evaluating Risk Factors Without Selection Bias in a Real-World, Population-Based Cohort From 2009 to 2019', Clinical Oncology (2022), DOI: 10.1016/j.clon.2022.03.015
- 'Dynamic Risk Prediction of 30-Day Mortality in Patients With Advanced Lung Cancer: Comparing Five Machine Learning Approaches', CO Clin Cancer Inform. (2022), DOI: 10.1200/CCI.22.00054